Authors

Tian Yi Xia1, Vlad Marinescu1, MinSeo Hur1, Ashwini Adhikari1, Theodore Philipe2, Jessica Liddell2
1Youreka Montreal, 2McGill University
Abstract
Antibiotic resistance (ABR) is a growing global health concern that threatens the future of human wellbeing. The leading causes of increased ABR—excessive and inappropriate use of antibiotics—can be linked to a diverse array of multidisciplinary environmental factors, largely those related to the healthcare, agricultural, and food industries. Here, we employ machine learning to understand antibiotic resistance trends, utilizing a dataset of 804 socioeconomic, environmental, and demographic indicators to identify the most effective predictors of and factors contributing to ABR, and predict antibiotic resistance for years with available data using the model. We predicted that machine learning would be able to accurately predict antibiotic resistance and that the significant predictors would be diverse, though primarily environmental-related. We found that global antibiotic use and resistance risk has predictables trends by means of machine learning, with our models’ accuracy being above 95%. We also found that the best predictors of ABR were most often environmental factors. Nonetheless, the significance of a diverse array of factors indicates that antibiotic use and resistance is an inherently multidisciplinary issue. Overall, our study can be used to inform policymakers across all disciplines to implement measures to mitigate the rise of antibiotic resistance.
Poster
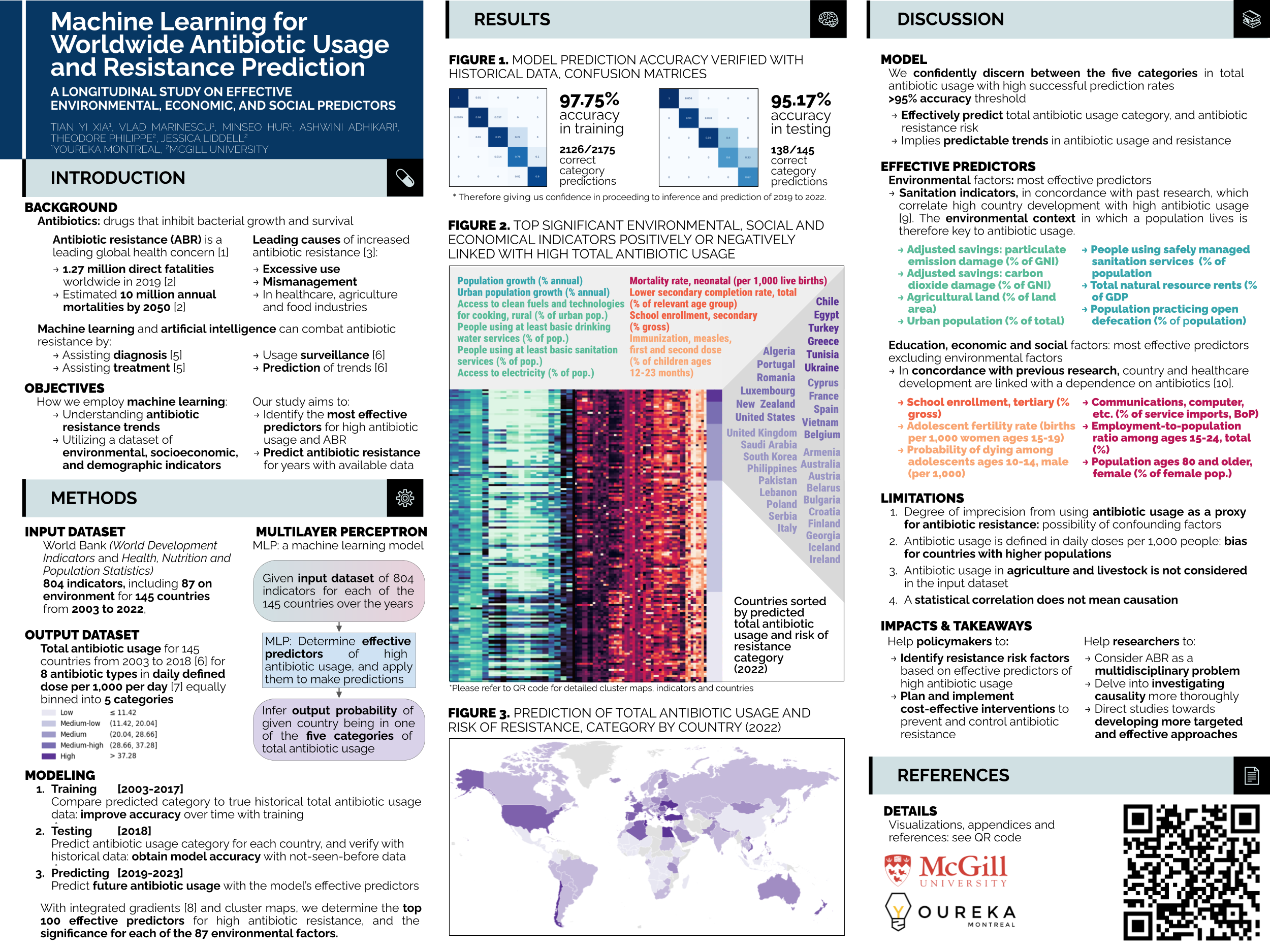
Results
Confusion matrices in predicting total antibiotic usage
Predicted and actual total antibiotic usage category confusion matrix for training data
(Click image to enlarge)

Predicted and actual total antibiotic usage category confusion matrix for testing data
(Click image to enlarge)

Correspondence between top significant indicators and total antibiotic usage
Worldwide analysis of 2022
Top significant indicators, all categories
- n=100 indicators
- n=145 countries
(Click image to enlarge)

Top significant indicators, environmental
- n=87 environmental and climate change indicators
- n=145 countries
(Click image to enlarge)

Prediction of total antibiotic usage and risk of resistance, category by country
(Click image to interact)

Paper
Machine Learning for Worldwide Antibiotic Usage and Resistance Prediction: A Longitudinal Study on Effective Environmental, Economical, and Social Predictors
Poster References
- [1] Murray, C. J. L., Ikuta, K. S., Sharara, F., Swetschinski, L., Aguilar, G. R., Gray, A., Han, C., Bisignano, C., Rao, P., Wool, E., Johnson, S. C., Browne, A. J., Chipeta, M. G., Fell, F., Hackett, S., Haines-Woodhouse, G., Hamadani, B. H. K., Kumaran, E. A. P., McManigal, B., … Naghavi, M. (2022). Global burden of bacterial antimicrobial resistance in 2019: A systematic analysis. The Lancet, 399(10325), 629–655. https://doi.org/10.1016/S0140-6736(21)02724-0
- [2] Tang, K. W. K., Millar, B. C., & Moore, J. E. (2023). Antimicrobial Resistance (AMR). British Journal of Biomedical Science, 80, 11387. https://doi.org/10.3389/bjbs.2023.11387
- [3] Lee, C.-R., Cho, I. H., Jeong, B. C., & Lee, S. H. (2013). Strategies to Minimize Antibiotic Resistance. International Journal of Environmental Research and Public Health, 10(9), Article 9. https://doi.org/10.3390/ijerph10094274
- [4] Sakagianni, A., Koufopoulou, C., Feretzakis, G., Kalles, D., Verykios, V. S., Myrianthefs, P., & Fildisis, G. (2023). Using Machine Learning to Predict Antimicrobial Resistance―A Literature Review. Antibiotics, 12(3), Article 3. https://doi.org/10.3390/antibiotics12030452
- [5] Kanjilal, S., Oberst, M., Boominathan, S., Zhou, H., Hooper, D. C., & Sontag, D. (2020). A decision algorithm to promote outpatient antimicrobial stewardship for uncomplicated urinary tract infection. Science Translational Medicine, 12(568), eaay5067. https://doi.org/10.1126/scitranslmed.aay5067
- [6] Browne, A. J., Chipeta, M. G., Haines-Woodhouse, G., Kumaran, E. P. A., Hamadani, B. H. K., Zaraa, S., Henry, N. J., Deshpande, A., Reiner, R. C., Day, N. P. J., Lopez, A. D., Dunachie, S., Moore, C. E., Stergachis, A., Hay, S. I., & Dolecek, C. (2021). Global antibiotic consumption and usage in humans, 2000–18: A spatial modelling study. The Lancet Planetary Health, 5(12), e893–e904. https://doi.org/10.1016/S2542-5196(21)00280-1
- [7] Defined Daily Dose (DDD). (n.d.). Retrieved April 9, 2024, from https://www.who.int/tools/atc-ddd-toolkit/about-ddd
- [8] Sundararajan, M., Taly, A., & Yan, Q. (2017). Axiomatic Attribution for Deep Networks (arXiv:1703.01365). arXiv. https://doi.org/10.48550/arXiv.1703.01365
- [9] Istúriz, R. E., & Carbon, C. (2000). Antibiotic Use in Developing Countries. Infection Control & Hospital Epidemiology, 21(6), 394–397. https://doi.org/10.1086/v501780
- [10] Klein, E. Y., Van Boeckel, T. P., Martinez, E. M., Pant, S., Gandra, S., Levin, S. A., Goossens, H., & Laxminarayan, R. (2018). Global increase and geographic convergence in antibiotic consumption between 2000 and 2015. Proceedings of the National Academy of Sciences, 115(15), E3463–E3470. https://doi.org/10.1073/pnas.1717295115